مدل زمین سه بعدی با استفاده از شبکه های عصبی با Python Scikit Learn و Vtk
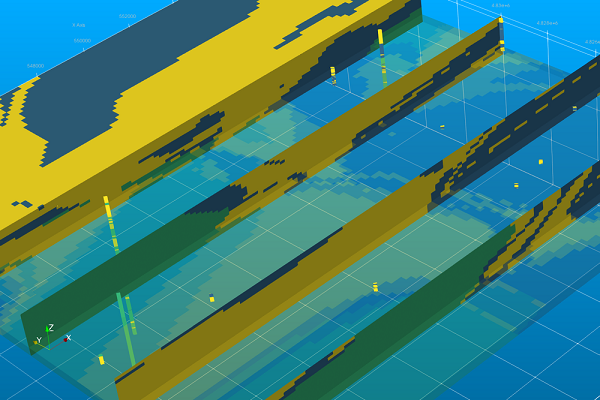
Scripts
This is the whole Python script used for the tutorial:
#import required libraries
%matplotlib inline
import os
import numpy as np
import pandas as pd
import matplotlib.pyplot as plt
import pyvista as pv
import vtk
Import well location and lithology
From the publicacion: https://pubs.usgs.gov/sir/2019/5138/sir20195138_v1.1.pdf The selected units would be:
- Coarse-grained fluvial and alluvial deposits
- Pliocene-Pleistocene and Miocene basalts
- Fine-grained lacustrine deposits
- Rhyolitic and granitic bedrock
wellLoc = pd.read_csv('../inputData/TV-HFM_Wells_1Location_Wgs11N.csv',index_col=0)
wellLoc.head()
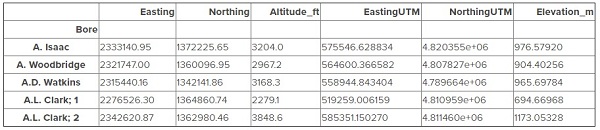
wellLito = pd.read_csv('../inputData/TV-HFM_Wells_2Lithology_m_rcl_clip.csv',index_col=0)
wellLito.head()

Point cloud of lithologies
litoPoints = []
for index, values in wellLito.iterrows():
wellX, wellY, wellZ = wellLoc.loc[values.Bore][["EastingUTM","NorthingUTM","Elevation_m"]]
wellXY = [wellX, wellY]
litoPoints.append(wellXY + [values.topLitoElev_m,values.hydrogeoCode])
litoPoints.append(wellXY + [values.botLitoElev_m,values.hydrogeoCode])
litoLength = values.topLitoElev_m - values.botLitoElev_m
if litoLength < 1:
midPoint = wellXY + [values.topLitoElev_m - litoLength/2,values.hydrogeoCode]
else:
npoints = int(litoLength)
for point in range(1,npoints+1):
disPoint = wellXY + [values.topLitoElev_m - litoLength*point/(npoints+1),values.hydrogeoCode]
litoPoints.append(disPoint)
litoNp=np.array(litoPoints)
np.save('../outputData/litoNp',litoNp)
litoNp[:5]
array([[5.48261389e+05, 4.83802316e+06, 7.70442960e+02, 1.00000000e+00],
[5.48261389e+05, 4.83802316e+06, 7.70138160e+02, 1.00000000e+00],
[5.48261389e+05, 4.83802316e+06, 7.70138160e+02, 3.00000000e+00],
[5.48261389e+05, 4.83802316e+06, 7.68614160e+02, 3.00000000e+00],
[5.48261389e+05, 4.83802316e+06, 7.69376160e+02, 3.00000000e+00]])
Coordinate transformation and Neural Network Classifier setup
from sklearn.neural_network import MLPClassifier
from sklearn.metrics import confusion_matrix
from sklearn import preprocessing
litoX, litoY, litoZ = litoNp[:,0], litoNp[:,1], litoNp[:,2]
litoMean = litoNp[:,:3].mean(axis=0)
litoTrans = litoNp[:,:3]-litoMean
litoTrans[:5]
#setting up scaler
scaler = preprocessing.StandardScaler().fit(litoTrans)
litoScale = scaler.transform(litoTrans)
#check scaler
print(litoScale.mean(axis=0))
print(litoScale.std(axis=0))
[ 2.85924590e-14 -1.10313442e-15 3.89483608e-20]
[1. 1. 1.]
#run classifier
X = litoScale
Y = litoNp[:,3]
clf = MLPClassifier(activation='tanh',solver='lbfgs',hidden_layer_sizes=(15,15,15), max_iter=2000)
clf.fit(X,Y)
C:\Users\Gida\Anaconda3\lib\site-packages\sklearn\neural_network\_multilayer_perceptron.py:470: ConvergenceWarning: lbfgs failed to converge (status=1):
STOP: TOTAL NO. of ITERATIONS REACHED LIMIT.
Increase the number of iterations (max_iter) or scale the data as shown in:
https://scikit-learn.org/stable/modules/preprocessing.html
self.n_iter_ = _check_optimize_result("lbfgs", opt_res, self.max_iter)
MLPClassifier(activation='tanh', alpha=0.0001, batch_size='auto', beta_1=0.9,
beta_2=0.999, early_stopping=False, epsilon=1e-08,
hidden_layer_sizes=(15, 15, 15), learning_rate='constant',
learning_rate_init=0.001, max_fun=15000, max_iter=2000,
momentum=0.9, n_iter_no_change=10, nesterovs_momentum=True,
power_t=0.5, random_state=None, shuffle=True, solver='lbfgs',
tol=0.0001, validation_fraction=0.1, verbose=False,
warm_start=False)
Determination of confusion matrix
numberSamples = litoNp.shape[0]
expected=litoNp[:,3]
predicted = []
for i in range(numberSamples):
predicted.append(clf.predict([litoScale[i]]))
results = confusion_matrix(expected,predicted)
print(results)
[[1370 128 377 0]
[ 67 2176 10 0]
[ 274 33 1114 0]
[ 1 0 0 151]]
Area of study and output grid refinement
xMin = 540000
xMax = 560000
yMin = 4820000
yMax = 4840000
zMax = int(wellLito.topLitoElev_m.max())
zMin = zMax - 300
cellH = 200
cellV = 20
Determination of the lithology matrix
vertexCols = np.arange(xMin,xMax+1,cellH)
vertexRows = np.arange(yMax,yMin-1,-cellH)
vertexLays = np.arange(zMax,zMin-1,-cellV)
cellCols = (vertexCols[1:]+vertexCols[:-1])/2
cellRows = (vertexRows[1:]+vertexRows[:-1])/2
cellLays = (vertexLays[1:]+vertexLays[:-1])/2
nCols = cellCols.shape[0]
nRows = cellCols.shape[0]
nLays = cellLays.shape[0]
i=0
litoMatrix=np.zeros([nLays,nRows,nCols])
for lay in range(nLays):
for row in range(nRows):
for col in range(nCols):
cellXYZ = [cellCols[col],cellRows[row],cellLays[lay]]
cellTrans = cellXYZ - litoMean
cellNorm = scaler.transform([cellTrans])
litoMatrix[lay,row,col] = clf.predict(cellNorm)
if i%30000==0:
print("Processing %s cells"%i)
print(cellTrans)
print(cellNorm)
print(litoMatrix[lay,row,col])
i+=1
Processing 0 cells
[-8553.96427073 8028.26104284 356.7050941 ]
[[-1.41791371 2.42904321 1.11476509]]
3.0
Processing 30000 cells
[-8553.96427073 8028.26104284 296.7050941 ]
[[-1.41791371 2.42904321 0.92725472]]
3.0
Processing 60000 cells
[-8553.96427073 8028.26104284 236.7050941 ]
[[-1.41791371 2.42904321 0.73974434]]
3.0
Processing 90000 cells
[-8553.96427073 8028.26104284 176.7050941 ]
[[-1.41791371 2.42904321 0.55223397]]
2.0
Processing 120000 cells
[-8553.96427073 8028.26104284 116.7050941 ]
[[-1.41791371 2.42904321 0.3647236 ]]
2.0
plt.imshow(litoMatrix[0])
<matplotlib.image.AxesImage at 0x14fb8688860>
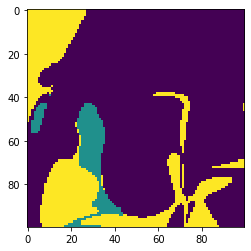
plt.imshow(litoMatrix[:,60])
<matplotlib.image.AxesImage at 0x14fb871d390>
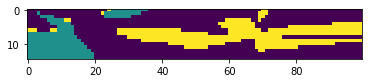
np.save('../outputData/litoMatrix',litoMatrix)
#matrix modification for Vtk representation
litoMatrixMod = litoMatrix[:,:,::-1]
np.save('../outputData/litoMatrixMod',litoMatrixMod)
plt.imshow(litoMatrixMod[0])
<matplotlib.image.AxesImage at 0x14fb87825f8>
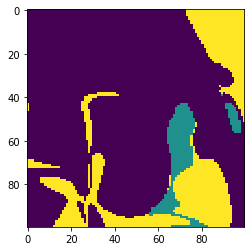
Generation of regular grid VTK
import pyvista
import vtk
# Create empty grid
grid = pyvista.RectilinearGrid()
# Initialize from a vtk.vtkRectilinearGrid object
vtkgrid = vtk.vtkRectilinearGrid()
grid = pyvista.RectilinearGrid(vtkgrid)
grid = pyvista.RectilinearGrid(vertexCols,vertexRows,vertexLays)
litoFlat = list(litoMatrixMod.flatten(order="K"))[::-1]
grid.cell_arrays["hydrogeoCode"] = np.array(litoFlat)
grid.save('../outputData/hydrogeologicalUnit.vtk')
شناسه تلگرام مدیر سایت: SubBasin@
نشانی ایمیل: behzadsarhadi@gmail.com
(سوالات تخصصی را در گروه تلگرام ارسال کنید)
_______________________________________________________
پروژه تخصصی در لینکدین
نظرات (۰)